tMRI Lab (Bickelhaupt)
Our “transformative oncologic imaging” (tMRI) Lab of the Institute of Radiology (Director: Prof. Dr. med. Michael Uder) at the University Hospital Erlangen conducts research in the field of transformative oncologic imaging and cancer prevention and combines research with innovative projects in the field of digital health.
One focus is on the systematic processing of complex MRI biosignatures in combination with artificial intelligence (AI) methods and the integration of additive data sources of digital health applications in oncology. We integrate our research projects into the entire oncological diagnostic process, from patient preparation, planning and predictive quality assurance in image acquisition to analysis in the context of interdisciplinary treatment processes, and are actively involved in national and international research networks in the field of digital health.
Our long-term vision is to improve oncological care through advances in translational diagnostic imaging. To this end, we are researching and developing new transformative methods that have the potential to make oncological imaging more available, personalized and predictive.
We are currently bundling our research activities in three research teams for the sections “AI in Oncologic Imaging”, “Digital Health” and “Innovation Networks, Data Science & Research Support”, and we also actively support the digital health innovation platform in Erlangen (d.hip).
Team “AI in Oncologic Imaging”:
Virtuelle Kontrastmittel für die MRT-Bildgebung
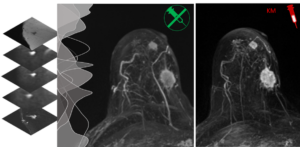
Virtual contrast agents for MR imaging
Many MRI examinations today require the intravenous injection of gadolinium-based contrast agents (GBCA). The combination of the latest MR imaging techniques, such as advanced diffusion sequences (DWI), with artificial intelligence (AI) methods could help to reduce the need for GBCA in MRI diagnostics in the future. The aim of our research is therefore to develop techniques for the virtual generation of contrast-analog image contrasts and to conduct translational research, with a focus on their use in breast cancer diagnostics and early detection.
Contact: Dr. Andrzej Liebert, Hannes Schreiter, Luise Brock, Shirin Heidarikakesh
Image quality in oncological MRI diagnostics
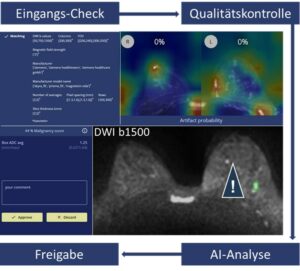
Advanced imaging techniques such as MRI pose technical challenges in their application, which can lead to image artifacts making it difficult for radiologists to make an assessment. In the future, artificial intelligence and machine learning techniques could help to detect image artifacts close to the scanner using smart technologies or even predict them during the examination procedure, thus creating opportunities for intervention. The aim of our research is to develop such techniques for breast cancer diagnostics.
Contact: Dr. Lorenz Kapsner,Luise Brock, Dr. Andrzej Liebert
New imaging methods for risk stratification in breast cancer screening
Traditionally, the aim of imaging in the early detection of cancer is to detect conspicuous changes and to be able to refer them for further treatment. Beyond lesion detection, personalized risk stratification is becoming increasingly important. Modern imaging techniques such as MRI may be able to make an important contribution in the future through multispectral and multidimensional biosignature analyses. Together with the MR physics working groups, we are therefore researching the potential of complex MR biosignature analyses in high and ultra-high field MRI.
Contact: Astrid Müller, PD Dr. med. Sebastian Bickelhaupt, MBA
Diffusion-weighted MRI in oncological diagnostics
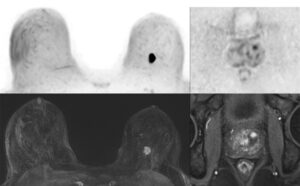
Diffusion-weighted MRI (DWI) maps the random movements of water molecules in the tissue. This provides an in-depth insight into the tissue microstructure, which can be used as complementary information in MRI to further support the diagnostic assessment. New DWI techniques are being evaluated in translational studies, e.g. in breast and prostate MRI.
Contact: Dominika Skwierawska, Dr. Dominique Hadler
_______________________________________________________________________________________
Team “Digital Health”:
Patient preparation for MR examinations using VR
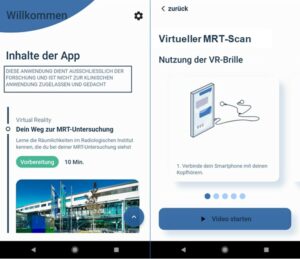
Diagnostic examinations such as MR imaging can be a stressful experience for patients. This is partly due to the unclear medical situation that makes diagnostics necessary, as well as the unknown nature of the examination. Digital solutions such as applications that implement virtual reality (VR) could become important tools in patient preparation. Together with partners in Germany and abroad, we are researching the development of corresponding procedures.
Contact: Sarah Seidnitzer
TEAM-X Inkubator – focused support for innovations
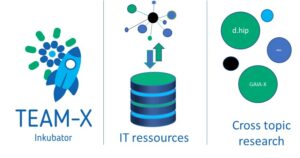
Developing digital applications from the concept idea to the first prototypes is often a major challenge for people from research and clinical practice. We have therefore established the TEAM-X Incubator as part of BMWK funding, which enables concept teams to develop and test their ideas for a digital healthcare solution. The work is embedded in the overall TEAM-X consortium, which is developing and researching new approaches to data sovereignty in the context of GAIA-X.
Contact: Dr. Judith Lach, Sarah Seidnitzer, Hannes Schreiter, Dr. Tobit Führes.
Trusted Ecosystem for Medical Data Exchange – GAIA-X
In this BMWK-funded project, GAIA-X-based technologies are evaluated in medical use cases. The aim is to develop technologies based on GAIA-X that can enable secure and sovereign data exchange in the healthcare sector, with the team coordinating the activities of the University Hospital Erlangen.
Contact: Dr. Judith Lach, Sarah Seidnitzer, Hannes Schreiter, Dr. Tobit Führes.
_______________________________________________________________________________________
Team „Innovation Networks, Data Science & Research Support“:
Interdisciplinary Co-Working Space
Transformative research thrives on interdisciplinary collaboration. For this reason, we have established and operate workstations in our lab that are specifically designed to provide space for interdisciplinary collaboration and exchange.
Contact: Hannes Schreiter, Dr. Andrzej Liebert
Data storage and structuring
Many research projects, especially in the fields of artificial intelligence (AI) and machine learning (ML), benefit from aggregated and structured data. Together with the Medical Center for Information and Communication Technology of the University Hospital, we are working to support the scientists in and around our own lab with specific IT infrastructure and to be able to analyze particularly high-quality curated data for the research projects in a targeted manner.
Contact: Dr. Lorenz Kapsner, Hannes Schreiter, Dr. Andrzej Liebert, Aju George, Dr. Dominique Hadler, Jessica Eberle, Chris Ehring
d.hip digital health innovation Plattform
The digital health innovation platform (d.hip) is a joint initiative of the hospital, university, industry and Medical Valley EMN to design new innovative projects in the field of digital healthcare and conduct translational research in scientific projects.
Contact: PD Dr. med. Sebastian Bickelhaupt, MBA
We are an integrative part of the oncological research groups of the Institute of Radiology (Big Data and Gynecological Imaging), the Radiology Department at the CCC Erlangen-EMN, actively cooperate with clinical and technical disciplines at the University Hospital and the University as well as nationally and internationally with research institutions such as the German Cancer Research Center Heidelberg (DKFZ) and actively participate in international networks and cooperation projects such as the RLS Sciences Digital Health Initiative of the Scientific Coordination Office of the Bavarian Research Alliance.
We would like to thank the German Research Foundation (DFG), the Federal Ministry of Education and Research (BMBF), the Federal Ministry of Economic Affairs and Climate Protection (BMWK), the Bavarian State Ministry of Science and the Arts, the Bavarian Research Alliance, the Bavarian Institute for Digital Transformation (bidt), the Else Kröner-Fresenius Foundation (EKFS) and the Dr. Oskar Dünisch Foundation, among others, for funding our research.